Expo
view channel
view channel
view channel
view channel
view channel
view channel
view channel
Medical Imaging
AI
Surgical TechniquesPatient CareHealth ITPoint of CareBusiness
Events
- Machine Learning Tool Identifies Rare, Undiagnosed Immune Disorders from Patient EHRs
- On-Skin Wearable Bioelectronic Device Paves Way for Intelligent Implants
- First-Of-Its-Kind Dissolvable Stent to Improve Outcomes for Patients with Severe PAD
- AI Brain-Age Estimation Technology Uses EEG Scans to Screen for Degenerative Diseases
- Wheeze-Counting Wearable Device Monitors Patient's Breathing In Real Time
- Novel Rigid Endoscope System Enables Deep Tissue Imaging During Surgery
- Robotic Nerve ‘Cuffs’ Could Treat Various Neurological Conditions
- Flexible Microdisplay Visualizes Brain Activity in Real-Time To Guide Neurosurgeons
- Next-Gen Computer Assisted Vacuum Thrombectomy Technology Rapidly Removes Blood Clots
- Hydrogel-Based Miniaturized Electric Generators to Power Biomedical Devices
- Surgical Capacity Optimization Solution Helps Hospitals Boost OR Utilization
- Game-Changing Innovation in Surgical Instrument Sterilization Significantly Improves OR Throughput
- Next Gen ICU Bed to Help Address Complex Critical Care Needs
- Groundbreaking AI-Powered UV-C Disinfection Technology Redefines Infection Control Landscape
- Clean Hospitals Can Reduce Antibiotic Resistance, Save Lives
- Mindray to Acquire Chinese Medical Device Company APT Medical
- Olympus Acquires Korean GI Stent Maker Taewoong Medical
- Karl Storz Acquires British AI Specialist Innersight Labs
- Stryker to Acquire French Joint Replacement Company SERF SAS
- Medical Illumination Acquires Surgical Lighting Specialist Isolux
- Strategic Collaboration to Develop and Integrate Generative AI into Healthcare
- AI-Enabled Operating Rooms Solution Helps Hospitals Maximize Utilization and Unlock Capacity
- AI Predicts Pancreatic Cancer Three Years before Diagnosis from Patients’ Medical Records
- First Fully Autonomous Generative AI Personalized Medical Authorizations System Reduces Care Delay
- Electronic Health Records May Be Key to Improving Patient Care, Study Finds
- AI Diagnostic Tool Accurately Detects Valvular Disorders Often Missed by Doctors
- New Model Predicts 10 Year Breast Cancer Risk
- AI Tool Accurately Predicts Cancer Three Years Prior to Diagnosis
- Ground-Breaking Tool Predicts 10-Year Risk of Esophageal Cancer
- AI Tool Analyzes Capsule Endoscopy Videos for Accurately Predicting Patient Outcomes for Crohn’s Disease
- Point of Care HIV Test Enables Early Infection Diagnosis for Infants
- Whole Blood Rapid Test Aids Assessment of Concussion at Patient's Bedside
- New Generation Glucose Hospital Meter System Ensures Accurate, Interference-Free and Safe Use
- Unique Quantitative Diagnostic System Enables Immediate Diagnosis and Treatment at POC
- POC Myocardial Infarction Test Delivers Results in 17 Minutes
Expo
view channel
view channel
view channel
view channel
view channel
view channel
view channel
Medical Imaging
AI
Surgical TechniquesPatient CareHealth ITPoint of CareBusiness
Events
Advertise with Us
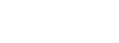
- Machine Learning Tool Identifies Rare, Undiagnosed Immune Disorders from Patient EHRs
- On-Skin Wearable Bioelectronic Device Paves Way for Intelligent Implants
- First-Of-Its-Kind Dissolvable Stent to Improve Outcomes for Patients with Severe PAD
- AI Brain-Age Estimation Technology Uses EEG Scans to Screen for Degenerative Diseases
- Wheeze-Counting Wearable Device Monitors Patient's Breathing In Real Time
- Novel Rigid Endoscope System Enables Deep Tissue Imaging During Surgery
- Robotic Nerve ‘Cuffs’ Could Treat Various Neurological Conditions
- Flexible Microdisplay Visualizes Brain Activity in Real-Time To Guide Neurosurgeons
- Next-Gen Computer Assisted Vacuum Thrombectomy Technology Rapidly Removes Blood Clots
- Hydrogel-Based Miniaturized Electric Generators to Power Biomedical Devices
- Surgical Capacity Optimization Solution Helps Hospitals Boost OR Utilization
- Game-Changing Innovation in Surgical Instrument Sterilization Significantly Improves OR Throughput
- Next Gen ICU Bed to Help Address Complex Critical Care Needs
- Groundbreaking AI-Powered UV-C Disinfection Technology Redefines Infection Control Landscape
- Clean Hospitals Can Reduce Antibiotic Resistance, Save Lives
- Mindray to Acquire Chinese Medical Device Company APT Medical
- Olympus Acquires Korean GI Stent Maker Taewoong Medical
- Karl Storz Acquires British AI Specialist Innersight Labs
- Stryker to Acquire French Joint Replacement Company SERF SAS
- Medical Illumination Acquires Surgical Lighting Specialist Isolux
- Strategic Collaboration to Develop and Integrate Generative AI into Healthcare
- AI-Enabled Operating Rooms Solution Helps Hospitals Maximize Utilization and Unlock Capacity
- AI Predicts Pancreatic Cancer Three Years before Diagnosis from Patients’ Medical Records
- First Fully Autonomous Generative AI Personalized Medical Authorizations System Reduces Care Delay
- Electronic Health Records May Be Key to Improving Patient Care, Study Finds
- AI Diagnostic Tool Accurately Detects Valvular Disorders Often Missed by Doctors
- New Model Predicts 10 Year Breast Cancer Risk
- AI Tool Accurately Predicts Cancer Three Years Prior to Diagnosis
- Ground-Breaking Tool Predicts 10-Year Risk of Esophageal Cancer
- AI Tool Analyzes Capsule Endoscopy Videos for Accurately Predicting Patient Outcomes for Crohn’s Disease
- Point of Care HIV Test Enables Early Infection Diagnosis for Infants
- Whole Blood Rapid Test Aids Assessment of Concussion at Patient's Bedside
- New Generation Glucose Hospital Meter System Ensures Accurate, Interference-Free and Safe Use
- Unique Quantitative Diagnostic System Enables Immediate Diagnosis and Treatment at POC
- POC Myocardial Infarction Test Delivers Results in 17 Minutes